Six Ways Your Kafka Design will Fail.
• • ☕️☕️☕️ 15 min readIn our blog series on data engineering and data science, we decided to use Kafka as a distributed data plane for the platform. For a fairly simple system, there is quite a lot to say about Kafka, and plenty of ways for you to embarrass yourself while implementing whatever business-value-adding-doovalacky is important this week. In this post we’ll cover some of the most common, dangerous, or strangest.
NB: I promise this will be the last conceptual post for a while and the next several will be pure implementation with substantially more code. While I personally think this post is a bit dry, I was explaining so many Kafka concepts in the following few that I decided to consolidate them all in a single post. I have tried to add some levity by swearing a lot, hopefully this helps.
Kafka Basics
Kafka is a key-value setup, where keys and values are understood only as arbitrary bytes. There are a few concepts to understand in order to use it effectively, including brokers, topics, partitions, replications and consumer groups. Kafka itself does relatively little, it doesn’t understand any data type other than raw bytes, makes no decisions about how to assign data across a cluster, and doesn’t decide how to feed that data back to consumers. Typically, you’ll be told that keys in Kafka are for either (a) partitioning/distributing the data on the cluster; or (b) something to do with indexing, like a database table index. Both statements are bullshit, and we’ll explore why below.
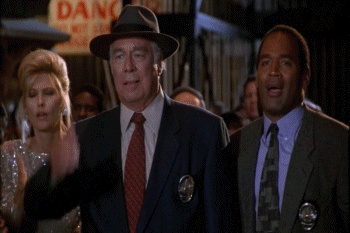
One of the reasons there might be so many misconceptions about the system is that there are a variety of other tools in the ecosystem which are often referred to in the same breath as Kafka (e.g. the Kafka streams library for Scala and Java, or the confluent Avro serialisers which interface with the schema registry - another component commonly deployed with kafka). All of these tools use Kafka’s primitives (partitions, topics, consumer groups) in different ways.
The schema registry in particular deserves a quick explanation, as it stores Avro schemata which are often used to serialise messages on Kafka. The schemata are required for both serialisation and deserialisation, and the schema registry is an external system which can provide these. The Confluent avro serialiser makes use of it to automatically retrieve and write schema in Java and Scala. Similar Kafka clients exist for Python also.
One further benefit of overprovisioning your partitions is that it helps with cluster rebalancing. Anyone who’s ever looked at discrete optimisation for any packing problem (or just taken an overseas flight on a budget airline) can attest that the most efficient optimisation is simply to make the components to be packed as small as possible. If we have 9 small partitions instead of 3 big ones, it becomes much easier to fit them onto brokers that might otherwise not have capacity for them.
Historically fewer partitions were desirable for performance reasons, but more recent versions of Kafka suffer few performance drawbacks from increasing the number.
Finally, it bears mentioning that different Kafka designs can make use of different partitioning arrangements. One common misconception (explored below) is that partitioning always happens to same way. Actually, it is the consumers and producers which decide how partitioning occurs. Kafka can map from partitions to brokers (and therefore retrieve the data in a particular partition) but it does not keep track of which data is in which partition.
Topics
Topics are the most visible entity in Kafka, so of course, everyone thinks they understand them. They are most usually wrong. Many of the misconceptions below are about topics, and those who repeat them are usually highly confident in their pronouncements.
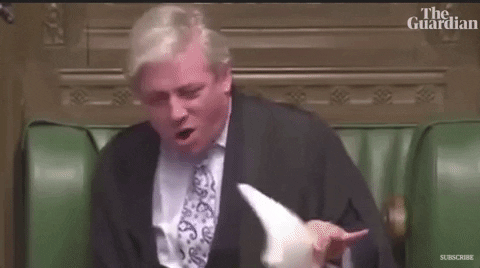
While there are analogies to be made between topics and database tables, and while we do often see that a topic will be linked to an Avro schema (and indeed, it might be only one schema, not several) none of these facts actually captures what a topic is for. In a nutshell, topics (at least in a well designed Kafka system) actually encapsulate an ordering of their messages.
Kafka is a streaming system, so delivery of messages in-order is important. Kafka guarantees that a given consumer will receive all messages on a given partition in the order they were written. There are two catches here -
- Messages between different topics enjoy no such guarantee.
- Message between partitions receive no such guarantee.
As a result, if you were in a situation where you wanted to preserve the ordering of a particular data stream within your system (for example, you’re implementing an event sourcing system), it needs to be mapped (through the partitioning strategy mentioned above) to a single partition. We give some examples below as to how this plays out later in this post, as messages being delivered out of order is one consequence of Kafka-fuck-ups numbers five through six, below. They are particularly interesting because solutions are not very widely available for all language APIs, and the documentation surrounding them is economical in its insights (if not outright nonsense and probably written by someone in marketing).
So why do we still talk about topics providing the ordering? Because topics hold both the partitions and the partitioning strategies, so provided the partitioning strategies are rational (and people tend not to think about these very much due to them often being provided by Confluent and the like) we can assume that we get the ordering guarantees we’re looking for.
Replications?
Replications differ from partitions, while partitions split data across the cluster to shard it out amongst the Kafka instances, replications copy the entire topic (with the same partitioning scheme) to other brokers. This is for durability and availability - so that broker failure cannot cause data loss - but one strange implication is that sometimes brokers will have the entire dataset on them, which seems at odds with the idea of using a distributed system. For example, if we have 3 brokers, partitions and replications, every replication has to be on a different broker, which means that each broker will have a replication of each partition. While this seems to defeat the point of using a distributed system, it is actually no different to the way striped RAID used to work, and once we add more brokers (if we decide we need to) we will find each partition of each replication (9 partitions) can be placed anywhere within the cluster.
Top misconceptions about Kafka
Ah the part you were waiting for… What are some of the ways all of this can go wrong? Normally things going wrong starts with someone misunderstanding how the system works and what’s implied by that. There are plenty of ways for this to happen even in a relatively simple system like Kafka.
1. Partitioning happens according to the key, therefore…
Actually partitioning happens according to whatever partitioning strategy our producers are using. You also want to hope that the consumers know about this, or they won’t know how to process the data they receive. I had this debate with some colleagues at one stage, where we discussed whether using differently named Avro schemata for a key would land messages on different partitions (because the hash of the key would be different due to the differing magic numbers in the two schemata).
From recollection, some further research turned up the fact that the Confluent Avro Serialiser actually does some work to hash the logical (not serialised) value of the Avro message, in order to correctly allocate it to a partition irrespective of the magic number, schema name, or other considerations.
2. All of our topics just have three partitions, there’s no point running more than three brokers…
The mistake seems obvious when you’re looking for it, but I’ve heard this more than once. Naturally, if you have more than one topic, you can distribute partitions for different topics to brokers across the cluster. Not every broker needs to hold the same set of topics.
So there you go, a bunch of ways to stuff up data systems based on Kafka. If you have seen anything else terrible, hilarious, or outright scary when developing systems on Kafka, let me know on Twitter and we can swap war stories.